OT Security for Digital Twins
The Project
Bridging the Gap
Enhancing Digital Twins with OT Security Insights
In Operational Technology (OT), the convergence of physical and
digital systems
represents a crucial step towards achieving greater efficiency, reliability, and safety in critical infrastructure and industrial operations. This year, I began a project that aims to do just that, not only by analyzing OT network traffic but also by leveraging machine learning techniques to build an innovative model for digital twins. Traditionally, digital twins have primarily relied on information fromICS/SCADA
systems. However, this approach misses out on the valuable data residing within Security OT monitoring systems like Intrusion Detection Systems (IDS). While the primary focus of these systems lies in security, they also harbor operational insights that conventional digital twin frameworks often overlook.Leveraging the Untapped Potential of OT Security Data
Intrusion Detection Systems (IDS) and other security monitoring tools excel at safeguarding network perimeters, but their potential to enrich digital twins with operational data remains largely unrealized. This is especially surprising considering their prime position for capturing valuable operational insights. While limitations like incomplete network visibility and performance versus protocol coverage trade-offs exist in real-time monitoring, the potential benefits outweigh these challenges.
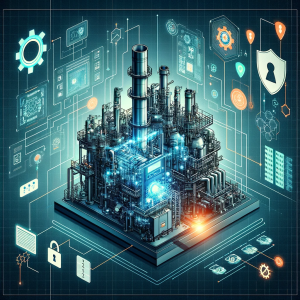

Unlocking the Challenges of OT Traffic Analysis
Extracting valuable insights from
OT traffic
presents two key challenges. First, the vast diversity of OT protocols creates a significant hurdle. While open-source libraries offer extensive protocol understanding, they often come at the cost of sacrificing system performance and resource efficiency. This creates a trade-off between comprehensiveness and practicality. Second, the demand for real-time or near real-time monitoring adds another layer of complexity. Deep protocol analysis can be crucial, but performing it without impacting system responsiveness becomes a balancing act. Finding the right balance between analysis depth and real-time performance is essential.Unveiling Deep Insights with Meticulous Extraction
To overcome the challenges of diverse protocols and real-time constraints, my project employs a deliberate approach I call “slow techniques” for data extraction. In contrast to real-time monitoring’s focus on speed, this method prioritizes depth and detail of protocol information. By meticulously dissecting OT protocols, I meticulously construct a dataset that feeds into machine learning algorithms with unprecedented granularity. This rich dataset acts as the foundation for a digital twin model that transcends simply reflecting the physical system’s current state. Empowered by the comprehensive insights gleaned from machine learning, the model becomes predictive and adaptive, offering invaluable foresight into potential issues and optimal operational adjustments.

Machine Learning: The Engine of Actionable Insights
Machine learning serves as the driving force behind this project, transforming the rich operational data into actionable insights. By harnessing its power, we expect to uncover hidden patterns, predict potential system failures with greater accuracy, and optimize operations in ways previously limited by data restrictions. This leap forward empowers us to proactively address issues, maximize efficiency, and ensure the smooth operation of critical infrastructure.
Open for collaboration
As I navigate through this project, the goal is not just to build a
digital twin
model but to redefine what digital twins can represent in the industrial sector. I have defined the challenges in which there some of which are open for collaboration, as follows:- How can digital twins improve predictive maintenance?
- What is the role of digital twins in product lifecycle management?
How do digital twins integrate with existing industrial systems?- What are the data requirements for a practical digital twin?
How can digital twins enhance operational efficiency?What are the cybersecurity implications of digital twins?- How can machine learning algorithms enhance the functionality of digital twins?
The targeted use cases in the next phase are the following:
- Predictive Maintenance and Condition Monitoring
- Process Optimization and Simulation
- Product Design and Development
- Asset Management
- Energy Management and Sustainability
- Supply Chain and Logistics Optimization
- Training and safety
While I am fortunate to contribute to the cybersecurity domain through my role at Forescout, this project is pursued independently during my weekends and free hours. It’s a labor of love and innovation, one that I believe can significantly impact our understanding and utilization of digital twins in OT environments.
I am eager to collaborate with like-minded professionals, researchers, and enthusiasts who share a passion for this cutting-edge intersection of OT, cybersecurity, and digital twin technology. Whether your expertise lies in machine learning, OT network protocols, cybersecurity measures, or any other relevant domain, your contribution can help steer this project towards groundbreaking discoveries and implementations.
How to Get Involved: If you’re interested in contributing to this project, please reach out to me at [email protected]. In your email, kindly include a brief overview of your background and specific areas where you feel you can contribute. I am looking for a diverse range of skills and perspectives to enrich this project, so don’t hesitate to share your unique insights and capabilities.